Data-backed triage: simplifying anti-money laundering alert management
In this article, we will delve into the concept of data-backed triage and explore how it can revolutionize anti-money laundering (AML) alert management.
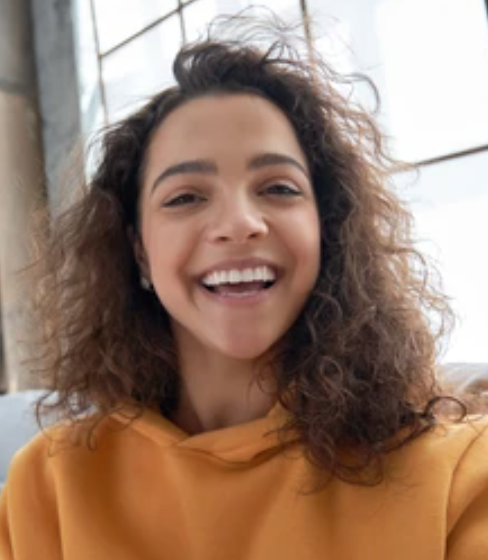
In this article, we will delve into the concept of data-backed triage and explore how it can revolutionize anti-money laundering (AML) alert management.
In today's fast-paced financial landscape, the battle against money laundering has become more complex than ever before. Financial institutions face the daunting task of sifting through an overwhelming number of alerts to identify suspicious activities while ensuring regulatory compliance. However, with the advent of data-backed triage, this arduous process can be streamlined and simplified. In this article, we will delve into the concept of data-backed triage and explore how it can revolutionize anti-money laundering (AML) alert management.
AML alerts are generated when a financial transaction or activity raises suspicions of potential money laundering or other illicit activities. Financial institutions are obligated to investigate these alerts and report any suspicious findings to the appropriate regulatory authorities. However, the sheer volume of alerts can be overwhelming, often resulting in high false-positive rates and limited resources being allocated to genuine threats.
Triage, a term borrowed from medical practice, refers to the process of prioritizing cases based on their severity and potential impact. Similarly, data-backed triage in AML alert management involves using advanced analytics and machine learning algorithms to categorize and prioritize alerts, reducing the burden on investigators and allowing them to focus on high-risk cases.
a. Historical analysis: By analyzing historical data, financial institutions can identify patterns and establish baseline behaviors. This allows them to separate routine transactions from potentially suspicious activities, reducing false positives.
b. Machine learning algorithms: Utilizing machine learning algorithms can enhance the efficiency of AML alert management. These algorithms can be trained to identify complex patterns and anomalies, enabling faster and more accurate identification of potential money laundering activities.
c. Risk scoring: Assigning risk scores to alerts based on various parameters, such as transaction size, location, and customer history, can help prioritize investigations. Alerts with higher risk scores receive immediate attention, while those with lower scores are subjected to automated or streamlined processes.
d. Collaboration and data sharing: Financial institutions can leverage shared data and collaborate with other organizations to enhance their AML efforts. Data sharing can help identify cross-institutional patterns and potential threats that individual institutions may overlook.
a. Improved efficiency: Data-backed triage streamlines the AML alert management process, allowing investigators to focus on high-risk cases promptly. This reduces the time and resources wasted on false positives, enabling more efficient allocation of investigative efforts.
b. Enhanced accuracy: By leveraging advanced analytics and machine learning algorithms, financial institutions can improve the accuracy of identifying suspicious activities. This reduces the risk of overlooking genuine threats while minimizing the number of unnecessary investigations.
c. Regulatory compliance: Implementing data-backed triage techniques ensures better compliance with AML regulations. By employing a systematic and data-driven approach, financial institutions can demonstrate their commitment to combating money laundering effectively.
d. Cost reduction: By optimizing alert management processes, financial institutions can reduce operational costs associated with AML compliance. Streamlining investigations and prioritizing resources help eliminate inefficiencies and unnecessary expenditures.
In an era where financial crime continues to evolve, financial institutions must embrace innovative approaches to combat money laundering effectively. Data-backed triage emerges as a game-changer in simplifying AML alert management. By leveraging historical data, advanced analytics, machine learning algorithms, and collaborative efforts, financial institutions can enhance efficiency, accuracy, and compliance while reducing costs. By staying at the forefront of technology and embracing data-driven practices, financial institutions can proactively safeguard their operations against money laundering threats.
Dataleon can help you bring your images and documents to life with ease.
Tester la plateforme gratuitementNous contacterTry 15 days
No credit card
Cancel Anytime